The Guardian Newspaper has an interesting article about the Pisa (Program for International Student Assessment) scores for 2012, and it includes data. Since I was interested to see how my own region scored, I downloaded the data into a file called PISA-summary-2012.csv
and created a plot summarizing scores in all the sampled regions, with Canada highlighted.
Summary graph, ranked in three categories
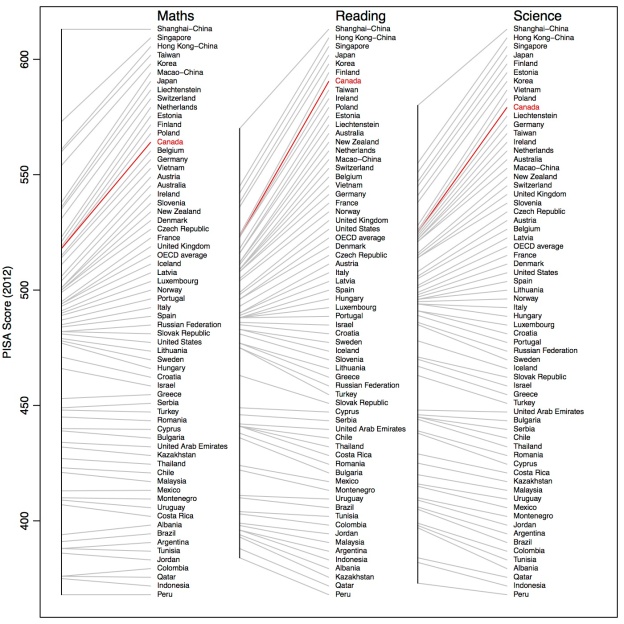
Summary of Pisa 2012 scores, broken down into category.
R code that creates the graph
The header length is unlikely to be the same in other years, nor the column names, so this code is brittle across similar datasets, but the necessary modifications for similar data should be obvious to anyone with passing familiarity with R.
regionHighlight <- "Canada"
d <- read.csv('PISA-summary-2012.csv', skip=16, header=FALSE,
col.names=c("rank","region",
"math","mathLow","mathHigh","mathChange",
"reading",'readingChange',
'science','scienceChange'))
n <- length(d$math)
par(mar=c(0.5, 3, 0.5, 0.5), mgp=c(2, 0.7, 0))
range <- range(c(d$math, d$reading, d$science))
plot(c(0, 6), range,
type='n', xlab="", axes=FALSE,
ylab="PISA Score (2012)")
axis(2)
box()
dy <- diff(par('usr')[3:4]) / 50 # vertical offset
x0 <- 0
dx <- 1
cex <- 0.65
## Math
o <- order(d$math, decreasing=TRUE)
y <- approx(1:n, seq(range[2],range[1],length.out=n), 1:n)$y
segments(rep(x0, n), d$math[o], rep(x0+dx, n), y,
col=ifelse(d$region[o]==regionHighlight, "red", "gray"))
lines(rep(x0, 2), range(d$math))
text(rep(x0+dx, n), y, d$region[o], pos=4, cex=cex,
col=ifelse(d$region[o]==regionHighlight, "red", "black"))
text(x0+dx, range[2]+dy, "Maths", pos=4, cex=1.2)
## Reading
x0 <- x0 + 2 * dx
o <- order(d$reading, decreasing=TRUE)
segments(rep(x0, n), d$reading[o], rep(x0+dx, n), y,
col=ifelse(d$region[o]==regionHighlight, "red", "gray"))
lines(rep(x0, 2), range(d$reading))
text(rep(x0+dx, n), y, d$region[o], pos=4, cex=cex,
col=ifelse(d$region[o]==regionHighlight, "red", "black"))
text(x0+dx, range[2]+dy, "Reading", pos=4, cex=1.2)
## Science
x0 <- x0 + 2 * dx
o <- order(d$science, decreasing=TRUE)
segments(rep(x0, n), d$science[o], rep(x0+dx, n), y,
col=ifelse(d$region[o]==regionHighlight, "red", "gray"))
lines(rep(x0, 2), range(d$science))
text(rep(x0+dx, n), y, d$region[o], pos=4, cex=cex,
col=ifelse(d$region[o]==regionHighlight, "red", "black"))
text(x0+dx, range[2]+dy, "Science", pos=4, cex=1.2)
Contents of the PISA-summary-2012.csv
data file
,,"Mean score
in PISA 2012, MATHS","Share
of low achievers
in mathematics
(Below Level 2)","Share
of top performers
in mathematics
(Level 5 or 6)","Annualised
change
in score points"," Mean score
in PISA 2012, READING","Annualised
change
in score points","Mean score
in PISA 2012, SCIENCE","Annualised
change
in score points"
1,Shanghai-China,613,3.8,55.4,4.2,570,4.6,580,1.8
3,Hong Kong-China,561,8.5,33.7,1.3,545,2.3,555,2.1
2,Singapore,573,8.3,40,3.8,542,5.4,551,3.3
7,Japan,536,11.1,23.7,0.4,538,1.5,547,2.6
12,Finland,519,12.3,15.3,-2.8,524,-1.7,545,-3
11,Estonia,521,10.5,14.6,0.9,516,2.4,541,1.5
5,Korea,554,9.1,30.9,1.1,536,0.9,538,2.6
17,Vietnam,511,14.2,13.3,m,508,m,528,m
14,Poland,518,14.4,16.7,2.6,518,2.8,526,4.6
13,Canada,518,13.8,16.4,-1.4,523,-0.9,525,-1.5
8,Liechtenstein,535,14.1,24.8,0.3,516,1.3,525,0.4
16,Germany,514,17.7,17.5,1.4,508,1.8,524,1.4
4,Taiwan,560,12.8,37.2,1.7,523,4.5,523,-1.5
20,Ireland,501,16.9,10.7,-0.6,523,-0.9,522,2.3
10,Netherlands,523,14.8,19.3,-1.6,511,-0.1,522,-0.5
19,Australia,504,19.7,14.8,-2.2,512,-1.4,521,-0.9
6,Macao-China,538,10.8,24.3,1,509,0.8,521,1.6
23,New Zealand,500,22.6,15,-2.5,512,-1.1,516,-2.5
9,Switzerland,531,12.4,21.4,0.6,509,1,515,0.6
26,United Kingdom,494,21.8,11.8,-0.3,499,0.7,514,-0.1
21,Slovenia,501,20.1,13.7,-0.6,481,-2.2,514,-0.8
24,Czech Republic,499,21,12.9,-2.5,493,,508,-1
18,Austria,506,18.7,14.3,0,490,-0.2,506,-0.8
15,Belgium,515,18.9,19.4,-1.6,509,0.1,505,-0.8
28,Latvia,491,19.9,8,0.5,489,1.9,502,2
-,OECD average,494,23.1,12.6,-0.3,496,0.3,501,0.5
25,France,495,22.4,12.9,-1.5,505,0,499,0.6
22,Denmark,500,16.8,10,-1.8,496,0.1,498,0.4
36,United States,481,25.8,8.8,0.3,498,-0.3,497,1.4
33,Spain,484,23.6,8,0.1,488,-0.3,496,1.3
37,Lithuania,479,26,8.1,-1.4,477,1.1,496,1.3
30,Norway,489,22.3,9.4,-0.3,504,0.1,495,1.3
32,Italy,485,24.7,9.9,2.7,490,0.5,494,3
39,Hungary,477,28.1,9.3,-1.3,488,1,494,-1.6
29,Luxembourg,490,24.3,11.2,-0.3,488,0.7,491,0.9
40,Croatia,471,29.9,7,0.6,485,1.2,491,-0.3
31,Portugal,487,24.9,10.6,2.8,488,1.6,489,2.5
34,Russian Federation,482,24,7.8,1.1,475,1.1,486,1
38,Sweden,478,27.1,8,-3.3,483,-2.8,485,-3.1
27,Iceland,493,21.5,11.2,-2.2,483,-1.3,478,-2
35,Slovak Republic,482,27.5,11,-1.4,463,-0.1,471,-2.7
41,Israel,466,33.5,9.4,4.2,486,3.7,470,2.8
42,Greece,453,35.7,3.9,1.1,477,0.5,467,-1.1
44,Turkey,448,42,5.9,3.2,475,4.1,463,6.4
48,United Arab Emirates,434,46.3,3.5,m,442,m,448,m
47,Bulgaria,439,43.8,4.1,4.2,436,0.4,446,2
43,Serbia,449,38.9,4.6,2.2,446,7.6,445,1.5
51,Chile,423,51.5,1.6,1.9,441,3.1,445,1.1
50,Thailand,427,49.7,2.6,1,441,1.1,444,3.9
45,Romania,445,40.8,3.2,4.9,438,1.1,439,3.4
46,Cyprus,440,42,3.7,m,449,m,438,m
56,Costa Rica,407,59.9,0.6,-1.2,441,-1,429,-0.6
49,Kazakhstan,432,45.2,0.9,9,393,0.8,425,8.1
52,Malaysia,421,51.8,1.3,8.1,398,-7.8,420,-1.4
55,Uruguay,409,55.8,1.4,-1.4,411,-1.8,416,-2.1
53,Mexico,413,54.7,0.6,3.1,424,1.1,415,0.9
54,Montenegro,410,56.6,1,1.7,422,5,410,-0.3
61,Jordan,386,68.6,0.6,0.2,399,-0.3,409,-2.1
59,Argentina,388,66.5,0.3,1.2,396,-1.6,406,2.4
58,Brazil,391,67.1,0.8,4.1,410,1.2,405,2.3
62,Colombia,376,73.8,0.3,1.1,403,3,399,1.8
60,Tunisia,388,67.7,0.8,3.1,404,3.8,398,2.2
57,Albania,394,60.7,0.8,5.6,394,4.1,397,2.2
63,Qatar,376,69.6,2,9.2,388,12,384,5.4
64,Indonesia,375,75.7,0.3,0.7,396,2.3,382,-1.9
65,Peru,368,74.6,0.6,1,384,5.2,373,1.3